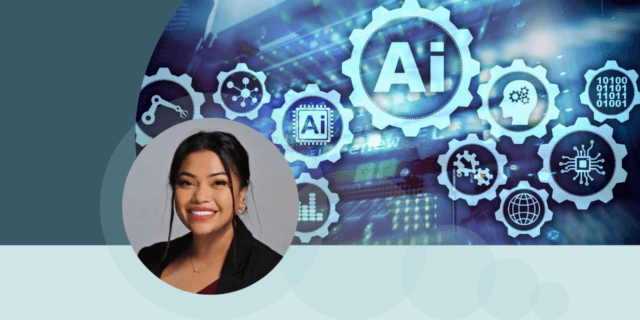
Leading into the Future: How AI is Revolutionizing Leadership Development
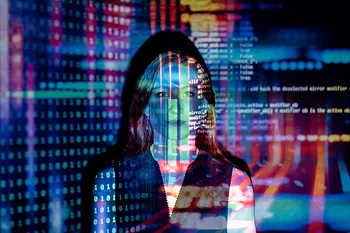
This white paper by Amanda Julian, PhD (Chief Science Officer and Co-Founder of Monark) examines the transformative impact of Artificial Intelligence (AI) and Machine Learning (ML) on leadership development, highlighting how these technologies can address shortcomings in traditional training methods. Traditional leadership training often struggles with infrequent and ineffective feedback, but AI offers a solution through real-time, actionable insights. By utilizing AI-powered role-play simulations and analyzing communications, leaders can receive immediate feedback, which is crucial for effective learning and application.
AI and ML also enable highly personalized learning experiences by tailoring training programs to individual needs, preferences, and proficiency levels. This personalization is achieved through sophisticated data analysis, which allows for dynamic and responsive training environments. Additionally, AI can enhance the application of new skills through targeted reminders and nudges, addressing common barriers such as forgetfulness and inertia that impede the transfer of learning to practical scenarios.
The integration of AI in coaching is another area of significant promise. AI-driven chatbots provide scalable, on-demand coaching, offering real-time, evidence-based advice tailored to individual challenges. This approach complements traditional coaching methods by increasing accessibility and efficiency.
However, the paper also stresses the importance of ethical considerations in the adoption of AI and ML. Issues such as data privacy, algorithmic bias, and transparency must be carefully managed to ensure that these technologies are used responsibly and effectively in leadership development. Balancing innovation with ethical responsibility is essential for maximizing the benefits of AI and ML in nurturing future leaders.